How Not to Do Mobile A/B Testing: 7 Fails of Mobile App Marketers
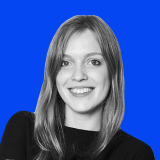
Split tests have become so mainstream that people are now launch A/B experiments for everything from educational videos to dating profiles (yes, this is happening). Yet, commoditization of the tactic means people too often fall into a trap of overlooking some basic rules A/B tests and experiments. Let’s look at some of the experiments fails in the context of mobile app store optimization.
It’s hardly possible to derive conclusions out of an experiment with fewer than 100 installs per variation. Smaller customers sample size will get you test results that are more folk wisdom than science. Obviously, a larger customers representative sample will yield more accurate data and improve your experience of the experiment. At the same time, you don’t want excessive responses, because driving mobile traffic to the test can be pricey.
You can use a few formulas to find out the best possible number of customers that should visit each variation of your experiment: from statistics to sample size calculation based on population size, margin of error, confidence error, and standard deviation. You can try a number of online tools to estimate the sample for your experiment, such as Stata, nQuery Advisor, and UnifyPow. The simplest way to calculate it online will perhaps be one of the following online tools:
SurveySystem Sample Size Calculator
When you run A/B tests with SplitMetrics, the software will track your experiment to ensure statistically significant results and enhancing your mobile app optimization experience.
When you run a test on a store page of your mobile app, it helps to be strategic about how you design the experiment. A solid optimization plan about what you’re going to test will keep you on track. A clear understanding of the desired A/B experiments outcome will get you to the goal faster and with fewer resources. Yet, before you formulate your A/B test hypothesis, ask yourself two questions:
1) What’s your app store optimization goal?
2) What could be a potential problem with a store page for your mobile app?
With app store page A/B testing, the goal is usually optimization of visitor-to-install conversion rate, and the problem is usually some design element(s) that could hinder it worsening customers app store page experience.
Now you can create a hypothesis which should be reflected in variations of your experiment. Two things should be in it: 1) what is your target improvement in conversion 2) what are you going to change to fix the problem you identified.
Let’s create a sample hypothesis for Rovio’s Angry Birds 2 app store page to illustrate coming up with variations for an optimization experiment.
If we consider an ever-increasing cost per click on mobile app install campaigns, even an incremental increase in conversion rate will help save a lot of money gaining new customers. A median conversion range for games, according to 2015 SplitMetrics comprehensive study on 10M users, is 4,47%. Let’s consider it our baseline conversion for a mobile app; and we’re feeling adventurous enough to try and get it to 10%, or a 5.53 points increase (+123,71%).
Here’s one idea: most mobile games developers publish app screenshots in landscape orientation in a store. For games category, it’s almost the norm, so – taking a guess here – if we switch to portrait mode, will our app store page stand out?
Mobile app conversion goal: app store page visitor-to-install conversion of 10%.
Problem statement: “Many games use landscape orientation screenshots, and ours doesn’t stand out”.
Hypothesis: “By switching screenshots of our mobile app store page from landscape orientation to portrait, we can achieve a higher conversion rate and optimization of customers experience”.
Now, with this hypothesis in mind, we can run tests on 2 sets of app store screenshots with portrait and landscape orientation.
If you use tracking tools such as Tune, Appsflyer, and Adjust, you can integrate them with your A/B experiments software and attribute new app’s customers to your ad campaigns. This will allow you to get rich data on customers you acquire and facilitate optimization process of ad channels.
SplitMetrics sends data to any mobile tracking or analytics software. To do so, we create a custom install URL for each A/B experiment you run by adding custom parameters. These parameters will be transferred to your app attribution partners so you could see how users who from different variations behave in the application.
When you run your first test, external factors may corrupt your experiments results. Iterative experiments come in handy not only to question and confirm overall conversion changes, but also to double-check which of the app store page elements triggered more installs and attracted more customers. You can focus on one app store page element (e.g., first screenshot, third screenshot, vs. read-more) and see if it was driving your campaign’s results.
The problem with multiple variations tests is that they are difficult to set up. They require extensive optimization planning and don’t really prove that a certain variation was responsible for the increase or decrease of app customers. With too many changes on your app store page at once, it’s hard to tell exactly what’s working.
Simple changes like tests of background color, orientation, a different first screenshot, and a different order of screenshots are much easier to set up and give app store optimization data that makes sense. If you don’t have the luxury of unlimited time and traffic, try to make one change at a time and then iterate.
Commit to a sample size before you start the experiment and wait until the test is over. I highly recommend reading Evan Miller’s article from back in 2010, as it’s one of the best online resources on the topic. Evan argues that A/B testing experiments used with a manual or automatic “stopping rule” will produce invalid tests.
This mistake is the result of what is known in statistics as “repeated significance testing errors”. Such sample size negligence spoils not only experiments results but your A/B testing experience as well
Seasonal buying habits of customers, demographic, market, product and competitor changes can all drive different results at various time points. To stay ahead of competition on the app market, you want to keep moving fast and be involved in app store optimization as you go.
Top mobile app publishers often take advantage of the multiple sales and promotions running on app stores. Such opportunities include Halloween-themed design changes in October, Christmas app store “decorations”, or even extreme app brand makeovers.
Here’s a list of additional online resources on A/B testing if you want to dig deeper into the topic and add this activity to your app store optimization strategy:
App store page A/B tests offer mobile marketers a bunch of benefits including higher conversion rate and an increase in organic app downloads from a store. The beauty of A/B experiments is that it’s like going straight to your customers and asking them what they like and don’t like about your app store page design.
However, with the rise of A/B testing cult comes the risk of overlooking the simple premises this scientific method is based on. We’ve covered 7 common mistakes marketers make when they conduct app store page split tests. By being strategic and smart about your app store optimization experiments, you’ll gain better and more reliable insights.