Sequential A/B Testing vs Multi-Armed Bandit Testing
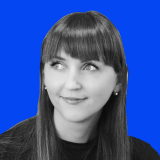
About a year ago SplitMetrics App Store A/B Testing Platform switched from classic A/B testing to the sequential approach. But even earlier we offered, and still do, an option: multi-armed bandit (MAB) testing. And we often hear a question: so, what is better, multi-armed bandit or sequential A/B testing? Well, that’s a tough question, since both MAB and the sequential A/B testing have their pros and cons, so every mobile publisher answers for themselves and chooses according to their business needs. However, to help you answer this question, we’ve created this post where you will find the key differences between the two methods embodied in the SplitMetrics platform, along with their strengths and use cases of each one, so that you could choose the approach that suits you best.
The essence of this method integrated in SplitMetrics app store A/B testing platform is that it compares a particular variation with one or multiple alternatives and determines the winner.
This is a universal and completely transparent approach that gives mobile publishers confidence that one of the variations performs better than the control – or vice versa.
In simple terms, with sequential A/B testing, the algorithm sends 50% of traffic to the control and 50% – to a variation (challenger). You run a test until you reach statistical significance, then get a winning variation and can apply your knowledge.
We do not make unfounded allegations about traffic. The amount of traffic that we show is the maximum amount of traffic, not the necessary one.
1. You are seeking to identify variations with statistical significance
Multi-armed bandit testing, which we will consider later on, is actually not the best choice if you are aiming to get a statistically significant winner. At the same time, sequential A/B testing experiments are the perfect way to get statistical significance you’re seeking.
For example, if you are at the prelaunch stage and still working on a new app or game, you might want to gather as much information as possible on the performance of your creatives, especially screenshots, to better understand which features are more important for your target audience, and further incorporate your learnings into your app.
2. You’d like to analyze the performance of all variations after an experiment is finished
Sequential A/B tests on SplitMetrics enable you to analyze how users behave on your app store product page, what elements draw their attention, whether they tend to scroll through your screenshots and watch app previews before pressing the download button or leaving the page.
Multi-armed bandit testing informs you what to do with traffic right now (it allocates traffic among all variations in the course of an experiment), while sequential A/B testing informs you what to do after an experiment: which variation (the winner) should be leveraged.
3. You strive to integrate findings from all variations to make critical business decisions
During an experiment, MAB sends most traffic to a variation that performs better than all others and sequential A/B testing, as we mentioned earlier, distributes traffic equally and shows you the winner, and this knowledge can be further integrated into your app store product page and utilized for other business purposes.
So if you need to collect data on all variations to make a crucial business decision, sequential A/B testing is what you are looking for.
To begin with, multi-armed bandit testing is not just one kind of testing – there is an entire class of algorithms.
The essence of multi-armed bandit testing is that, unlike sequential approach based on testing of statistical hypotheses, it continuously learns from data during experiments and increases the amount of traffic for better-performing variations, while decreasing for the underperforming ones. MAB adjusts to the environment over the whole test cycle.
Multi-armed bandit testing makes sure that during an experiment, the number of conversions will be maximized. By focusing on improving conversions, it moves statistical significance to the background.
The main focus of multi-armed bandit testing lies on optimizing overall conversions, while sequential A/B testing is aimed at finding out the performance of all variations.
However, as we mentioned before, there are many MAB algorithms. If we talk about SplitMetrics, multi-armed bandit testing is actually statistically robust. We at SplitMetrics use an algorithm called Thomas Sampling (or, Randomized Probability Matching). This method is equivalent to a Bayesian multi-armed bandit approach.
While sequential A/B testing names a winner, which is undoubtedly a big plus, multi-armed bandit testing implemented in SplitMetrics allocates traffic among alternatives in an optimum way. It estimates the conversion rate for each variation and distributes traffic accordingly: the better the conversion rate, the more traffic a variation gets.
1. You’re seeking to maximize conversions
If your main goal is to optimize the conversion rate and you don’t need to know the performance of each variation, multi-armed bandit testing with SplitMetrics is a perfect choice for you.
2. You’re optimizing for a time-limited offer (seasonal testing)
For example, if you’re testing creatives with new features dedicated to Halloween or Christmas, and offer some bonuses to users who will download your game or app during this period, you don’t have much time for gathering statistical significant results, so it is better to resort to MAB.
3. You are limited in the amount of traffic
With sequential A/B testing, you need as much traffic as it takes to get statistical significance. In case you don’t have enough traffic, multi-armed bandit testing will help you identify better performing variations much quicker, and these variations will get most of the traffic.
4. You are targeting your specific audience
Multi-armed bandit is a fast learner which applies the targeting rules you’ve specified to users that better fit your common audience, while continuing to experiment with less common audiences.