A/B/n Testing: Choose the Right Type of Experiment with SplitMetrics
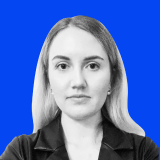
Unleash the full potential of A/B testing with core statistical methods based on your experiment goals with the advanced SplitMetrics experience.
SplitMetrics Optimize offers three methods within one platform.
No more gut feeling in running experiments. Growth, UA, and marketing managers can flexibly manage experiments and choose the most efficient A/B/n methods to skyrocket CRO or check concepts and hypotheses depending on goals. SplitMetrics’ comprehensive solution and dedicated team of experts will help you choose the best and most efficient way to run your experiments.
Using a spade for some jobs and a shovel for others does not require you to sign up to a lifetime of using only Spadian or Shovelist philosophy or to believe that only spades or shovels represent the One True Path garden neatness. There are different ways of tackling statistical problems, too.
The Bayesian testing is a new enhanced approach in experiments provided by SplitMetrics.
The Bayesian approach can be helpful in cases where marketers have some beliefs and knowledge to use as a primary assumption (in our case, it is informed prior in the default settings) that helps algorithms calculate the probability of related events to the likelihood of a specific outcome. Hence, users can make faster decisions with lower costs of experiments by incorporating beliefs or knowledge as part of the experiment, compared with the Frequentist or Sequential methods.
The benefits of the Bayesian approach in SplitMetrics are that users can find indirect control over the risk because they can control the expected loss stopping rule (threshold of caring).
The stopping rule – stops the test and reduces budgets once the winner or underperformer is obvious.
A Bayesian test starts from a weak prior assumption about the expected conversion (prior distribution). The prior may fix the noise at the beginning of the test when the sample size is small. As the test progresses and more visitors participate, the impact of the prior fades.
Leverage the Bayesian tests for growth hacking and iterative A/B testing if you need quick results with less traffic required.
Otherwise, if you don’t meet all of the criteria in your growth-hacking model, you’d better use the Sequential method.
There is a chance of getting stuck with a slight change (deterioration). Hence, it can accumulate a significant effect over time.
The possible flaws of Bayesian in some contexts are the side effects of treating control and test groups equally and controlling for cost/value rather than False Positive/False Negative error rate.
A conservative strategy is rationally justified
If you want a strict rule limiting the team’s discretion, you should try the Sequential approach with a standard p_value threshold.
Even if there is a slight difference between variations in the Bayesian approach, the one that looks at least a little better at the moment will be selected as recommended. Lean towards a conservative strategy with the Sequential approach that ensures significant statistical data for each variation to make conclusions for your global and high-cost-to-implement ideas.
Online cost optimization
Early stopping by toc (threshold of caring) with the expected loss tolerance if there’s an overperformer, underperformer, or approximately equal with the opportunity to spend fewer budgets on a test.
The Frequentist test came from the academic setup, where a false positive result is a major failure by default: you mislead the entire scientific community by reporting one. Thus, you are conservative by default: you prefer sticking to the current state and sacrificing exploitation for the low chance of being even marginally wrong.
Interpretability
The Bayesian A/B testing in the SplitMetrcis platform showcase a winning probability for experiment interpretability.
Take advantage of Bayesian tests for growth hacking and iterative A/B testing if you need quick results with less traffic required. Contact our experts to start your experiments with SplitMetrics.
A multi-armed bandit approach allows users to dynamically allocate traffic to perform well, while distributing less traffic in real-time to underperforming variations. This testing approach produces faster results since there’s no need to wait for a single winning variation.
This approach helps users effectively convey experiments with many variations while saving costs.
Marketers and product managers usually choose this type of A/B testing because of the smooth transition between exploration and exploitation, speed, and automation. It is more relevant for experiments with many variations. The SplitMetrics platform offers up to 8 variations per experiment. The automatic allocation of traffic minimizes the costs of experiments with no need to spend more budget for ineffective variations. By eliminating unnecessary and weak options, users simultaneously increase the likelihood of finding the most efficient option.
Bandit algorithms are relevant for short tests while allowing you to adjust in real-time and send more traffic more quickly for better variation. It’s applicable for seasonal promo campaigns and one-time promotions to choose the best option for budget allocation promptly.
Be careful when the algorithm sends more traffic to higher-performing content. It is likely to reinforce slight differences in low-traffic experiments and skewed results. There is a risk to considering a non-optimal option as optimal because there is less traffic: the fewer data, the less reliable the estimate.
Online cost optimization
Flexibility/Controls
Guarantees
Try the enhanced A/B/n testing experience with SplitMetrics.
The object of sequential sampling is to reduce the sample size, which must be drawn to decide on a population within specified error limits.
“Sequential sampling allows the experimenter to stop the trial early if the treatment appears to be a winner; it therefore addresses the “peeking” problem associated with eager experimenters who use (abuse) traditional fixed-sample methods. The above procedure can, in some circumstances, reduce the number of observations required for a successful experiment by 50% or more. The procedure works extremely well with low conversion rates (with small Bernoulli parameters). It works less well with high conversion rates, but traditional fixed-sample methods should do you just fine in those cases.”
– Evan Miller
Sequential test designs allow users to analyze data while the test runs to determine if an early decision can be made. Applicable for experiments with lower conversions. Done incorrectly, this is known as “peeking” and increases the risk of false-positive/negative errors. Relevant for experiments where you need to determine the exact significance level. You can analyze the performance of all variations after an experiment is finished.
Sequential A/B tests by SplitMetrics enable you to analyze how users behave on your app store product page, what elements draw their attention, and whether they tend to scroll through your screenshots and watch app previews before pressing the download button or leaving the page.
Relevant for testing global ideas on pre-launch, more applicable for massive experiments. Can reduce the chance of mistakes to reach the max. approximate solution.
Online cost optimization
Early stopping – works better with lower basic conversions (on higher conversions we need more traffic than in the classical Frequentist approach)
The calculations by Evan Miller demonstrate that:
1.5 x Baseline Conversion + Minimum Detectable Effect
Interpretability
Flexibility/Controls
Guarantees
Start using Sequential testing to check your high-cost global ideas with the SplitMetrics platform and dedicated team of experts.