A Practical Guide to Multi-Armed Bandit A/B Testing
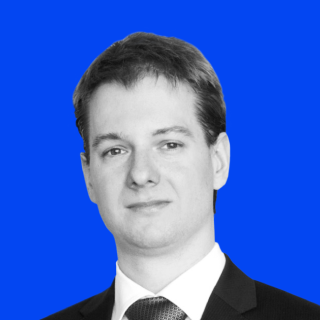
Mobile marketers, user acquisition specialists, app store optimization experts, app growth hackers – we are a practical bunch. We’re expected to deliver best results with limited resources in a short period of time (sometimes for yesterday ;) ).
Math can be fun and being a marketer requires knowing math. However, in an industry reliant on many mart-tech platforms, most math is performed under the hood. Automation rules. Smart scripts and AI help fish out key information and make smart decisions.
How Can AI Be Used In Mobile Marketing? AI tools, such as ChatGPT, are set to improve app marketers’ daily efforts by automating tedious tasks, generating content ideas, and allowing for more personalized user experiences. Read more about it in:
Some of us like to take a peek under the hood and this article is just for you. We’ll give you practical tips on using the multi-armed bandit testing on our platform (with examples), but for the happy data scientist in all of us we’ve included directions to some truly brainy papers. Enjoy!
Let’s start with the basic theory, that even the most die-hard practitioners should know:
In probability theory and machine learning, the multi-armed bandit problem is a problem in which a fixed limited set of resources must be allocated between competing (alternative) choices in a way that maximizes their expected gain, when each choice’s properties are only partially known at the time of allocation, and may become better understood as time passes or by allocating resources to the choice. Source: Wikipedia
Simple enough, but how does it translate to practical A/B/n (multi-variation) testing of mobile apps?
No rocket science here yet (that will come later in the article). Running tests of various iterations of our app’s product page costs some money. You just need to buy traffic (with Apple Search Ads, Facebook Ads, Google Ads and so on) to gather a sample population of users big enough to give you meaningful results.
There are situations where you need to be extra careful and shortcuts aren’t an option. Bad decisions during the design or pre-launch phase of developing and publishing an app can snowball into a disaster.
There are situations where fast results are needed and a larger margin of uncertainty is acceptable: when you need some experiments before launching a seasonal promotion, a timed event and so on.
SplitMetrics Optimize is a platform for running A/B tests and validating ideas. It offers three methods of testing: Bayesian, sequential and a multi-armed bandit to address varying business goals of app publishers. From their perspective, everything is automated. They can simply follow our validation framework and all math is done by the system. The whole focus of specialists is on the creative side: ideation, preparation of variants for testing, graphic design and so on.
This is how most of us marketers work. We understand WHAT is our goal and WHAT we want to accomplish and WHY we’re choosing a specific method of testing. We might as well sign up for a demo of SplitMetrics Optimize at this point to get to the neat stuff.
Okay, but WHY is the multi-armed bandit best suited for testing lower-priority changes on a short budget? Because of its key benefits:
The multi-armed bandit can deliver meaningful results in a relatively short period of time, allowing for real time adjustments, either manually or automatically.
This translates directly to the lower cost of the entire testing process. Quicker results and flexibility in ending the test means less money spent on ads that generate traffic.
These two benefits come with the relatively lower confidence level, which is acceptable for the changes the methodology is best for. But why is that and how does that work in practice?
It’s very simple: because the testing system will send more traffic to the best performing variation (after a warmp-up stage of 250 visitors per variation), spending your precious ad money on the one most likely to succeed, allowing you to halt the test entirely when you’re satisfied with results.
The key interpretability metric in the case of a multi-armed bandit test is “Weight”. Here’s a test results page from SplitMetrics Optimize:
The platform begins allocating weight only after a threshold of 250 visitors per variation is met. You can stop at this point (in case of a clear winner) or do the following:
After the warm-up period the best performing variation starts receiving more traffic than others. It helps to collect more traffic to the best performing variation in a short period of time and check whether the variation continues to perform well on a bigger sample size. That’s why Multi-armed-Methodology is best for seasonal experiments.
Imagine, you are in a casino. There are many different slot machines, from the so-called ‘one-armed bandits’ (an adequate name, as they are known for robbing players), to pachinkos and many others..
They are similar and do the same thing, but offer varying odds at winning. Some slot machines pay out more frequently than others.
If you knew beforehand which machine had the highest payoff, you would play that one exclusively to maximize your expected return from playing. The difficulty is that you do not know which slot machines have the best odds of paying out money..
Your resources (coins in your pocket) are limited. Your goal is to walk out of the casino with as much money as possible and not die there of old age.
The problem is to find the slot machine that is the best and get you the most money in the shortest amount of time.
It’s simple: after a certain number of tries, you start spending more money on the one machine that seems to be working best for you.
The math behind the multi-armed bandit approach is hard. It’s so hard in fact, that many approximate heuristic solutions are used for it. A good example will be the Thompson sampling heuristic.
Thompson sampling is an algorithm for online decision problems where actions are taken sequentially in a manner that must balance between exploiting what is known to maximize immediate performance and investing to accumulate new information that may improve future performance. Source: A Tutorial on Thompson Sampling, from the website of Stanford University.
Each of the multi-armed bandit experiments may require a specific bandit algorithm. For all the multi-armed bandits (data scientists or math enthusiasts, sorry ;) ) we recommend reading this paper: A modern Bayesian look at the multi-armed bandit, as it gives much insight into them.
Let’s start with a simple summarization, we’ll focus on testing methods available on the SplitMetrics Optimize platform.
In a nutshell:
Bayesian | Sequential | Multi-armed bandit | |
---|---|---|---|
Best for | Live apps, periodic & minor changes | Pre-launch, big changes & ideas | Seasonal experiments |
Traffic allocation | Randomly & equally | Randomly & equally | More to the best variation |
Interpretability | Improvement level, winning probability, chance to beat control | Improvement level, confidence level | Weight |
Key benefit | The golden middle methodology | High confidence | Speed & low cost |
As a consequence, practical differences in day-to-day work with these tests in SplitMetrics Optimize is as follows:
Expected confidence level can be adjusted for the sequential method, meaning that the sequential method can yield reliable information — for a price of necessary traffic to accomplish them.
The minimal number of visitors is significantly higher for the sequential method, translating directly to its higher cost. Ultimately, the multi-armed bandit is the cheapest method, having the lowest visitor threshold requirements.
Just for easy comparison, below you’ll find some more insight into these two additional methods.
The Bayesian approach is an industry gold standard (and our personal top choice) for iterative A/B testing and growth hacking, requiring less traffic. In Optimize, traffic is allocated equally and randomly between all alternatives. It’s the best methodology for live apps, validation of minor and periodical changes, as well as follow-up tests. Interpretability metrics are: improvement level relative to control, winning probability and probability to beat control.
The sequential approach is relevant for testing global ideas in pre-launch and soft-launch stages and more applicable for massive experiments where you need to determine the exact significance level. Can reduce the chance of mistakes to reach the max. approximate solution. It’s the best option for the pre-launch and soft-launch apps, as well as for live apps. With this method the system allocates traffic randomly and equally. Interpretability metrics are improvement level and confidence level.
It’s worth checking a great post discussing this issue: “When to Run Bandit Tests Instead of A/B/n Tests”. Generally speaking, specialists recommend using the multi-armed bandit methodology when you care about optimization. This method tends to work well for short tests (when you have a small amount of time) and paradoxically – long (ongoing) ones.
Let’s visit the App Store for a while. Let’s assume we’re testing headlines for Christmas versions of our apps. It’s the perfect use case for multi-armed bandit tests as time is essential here and these tests can determine quickly which headline is better.
If you are preparing your App Store product page for Christmas Holidays), you don’t want to spend an entire week exploring all the options, because once you learn anything, it’ll be too late to exploit the best variation.
Multi-Armed Bandit algorithms can be easily automated, that is why they are effective for long tests that constantly evolve. For example, if we need to determine the best order to display screenshots on our app’s product page regularly, it’s convenient to automate this task with a multi-armed bandit test.
Another long-term use of multi-armed bandit algorithms is targeting. Some types of users may be more common than others. Contextual multi-armed bandit testing can take advantage of this, by applying the learned targeting rules sooner for more common users, while continuing to learn (test) on the rules for the less common user types.
When it comes to mobile A/B testing, multi-armed bandit may become a real saver of marketing budget and time. If you launch your experiments with SplitMetrics A/B testing platform, it’s possible to activate this method, leave all concerns regarding formidable sample size behind and enjoy statistically significant results without extra expenses.
All methods have their purpose. The market’s golden standard is the Bayesian methodology, but depending on the market and available budgets, the multi-armed bandit might gain more prominence, as a cost-effective testing method.