Valid Mobile A/B Tests: Hypothesis, Traffic and Results Interpretation
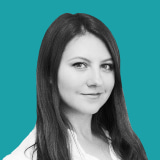
If you want your app conversions to boost overnight as if touched with a wand, you’ll be really disappointed. Anybody who is engaged in App Store Optimization will tell you that there’s no magic pill that will guarantee app’s stellar performance.
However, no matter how tempting it is to claim that the conversion rate is beyond your control, it’s vital to understand that a solid ASO strategy can help you get impressive results. Smart A/B testing should be an indispensable ingredient of such strategy.
ASO by no means should be limited to keywords optimization only. App publishers should never forget about the importance and app conversion impact of such product page elements as screenshots, icon, video preview, etc. After all, an app store page is the place where final decision-making takes place.
Mobile A/B testing of various product page elements is a really efficient way of optimizing your app’s conversion rate.
But remember that running your tests mindlessly will give no results, that’s why you should take into consideration quite a few aspects which predetermine success or failure of your experiments.
In this post, you’ll learn how to run valid a/b tests which can become conversion game changers.
If you are not ready to spare time for a thorough preparation, the chances of your experiment’s success shrink to zero. So it definitely makes sense to set aside some time for
The better you do your homework, the more chances your test will give meaningful results. So take enough time to do the research and analyze the market, competitors, target audience, etc.
You can start with the following steps:
Another aspect you should think over properly is your target audience. Having a clear portrait of your ideal users is a must. You should know everything about them from basic things like age and sex to their interests and preferences.
These insights will help to adapt your future app store creatives and ad campaigns. By the way, constant A/B testing helps to perfect your target audience portrait if you create ad groups with different audience settings to check which one performs better.
The research stage also includes the qualification of traffic sources. It’s really important to discover ad channels that bring more loyal users. You can use A/B testing for such qualification as well launching campaigns with the identical targeting in different ad networks and comparing their performance later.
Determining the most effective ad channel doesn’t only makes your A/B experiments more meaningful, this knowledge is essential for any marketing campaign in general.
According to the experience of our clients, Facebook and Adwords proved themselves as the best traffic sources while other ad channels have major shortcomings such as:
Only at fulfilling the above-mentioned steps, you can proceed to hypothesis elaboration. It is impossible to overstress the importance of a solid hypothesis for A/B testing.
Keep in mind that thoughtless split-experiments are doomed from the very beginning. A/B tests for the sake of A/B tests make absolutely no sense. So it definitely makes sense to take time and mull over a solid hypothesis worth proving.
But what makes a good hypothesis?
Remember that hypotheses are bold statements, they can’t be formulated as open-ended questions. A neat hypothesis should say what you plan to learn from an A/B experiment. Any A/B testing hypothesis should contain the following elements:
Here is an example of a good hypothesis formulated based on the abovementioned model:
If I change the orientation of the screenshots from horizontal to vertical, my app will get more installs as my target users are not hardcore gamers and are not used to landscape mode.
Keep in mind that it’s useless to A/B test practically identical variations. Don’t expect stellar results running an experiment for two icons for your game depicting the same character with different head posture.
What can be tested in this case then?
Various characters.
Background color.
Character’s facial expression, etc.
Let best practices you found out during the research be your inspiration for the test you can run.
A solid hypothesis will become a basis for further goals setting and choosing the experiment type. For instance, you decided to test screenshots orientation. Now you have to decide what kind of screenshots experiment you’d like to launch:
The results of such test will help to boost overall conversion of your product page. It’s extremely important if you mainly rely on high volumes of paid traffic.
This experiment type will show how your screenshots perform next to competitors. It’s essential for apps which stick to organic traffic and strive for better discoverability.
Now you are ready to design variations for testing and launch an A/B experiment. Thanks to SplitMetrics intuitive dashboard, starting a test is a matter of minutes.
It is essential to understand that no A/B experiment succeeded without decent and well-considered traffic. The truth is that you may have awesome creatives and use the best A/B testing platform but the poor quality and insufficient volume of traffic will predetermine the failure of your A/B experiment.
Running an A/B test, you should reach a really high confidence level before considering the experiment finished. If your test reaches 85% confidence, the system indicates the winner providing you have at least 50 installs per variation. Confidence is the statistical measurement used to evaluate the reliability of an estimate. For example, 97% confidence level indicates that the results of the test will hold true 97 times out of 100.
How much traffic do you need to run a meaningful experiment? Lots of publishers ask this question. Unfortunately, nobody will tell you the gold standard number which will guarantee high confidence level.
The necessary volume of traffic (mobile A/B testing sample size) is highly individual and depends on myriads of factors such as:
According to SplitMetrics observations, it normally takes at least 50 ‘Clicks to Install’ per variation to get statistically significant results.
On average, you need 400-500 visitors per variation for a test on your app’s product page and 800-1000 users per variation if you run an experiment on the search or category page. These numbers can help you take your bearings, but again, this index is highly individual.
It is better to use one of the specialized calculators to estimate a sample size of traffic for A/B testing. For example, one by Optimizely.
As was mentioned before, all factors are interconnected and require your close attention. However, one of the most impactful things you should consider is targeting.
Smart targeting helps to get statistically significant results buying less traffic. That’s why it’s so important to study your primary audience in the course of the research phase. The more granular your targeting, the better results you get. Lots publishers prefer to drive traffic from Facebook due to its amazing targeting options.
Read also: Facebook App Install Ads
To ensure trustworthy and quality test results, SplitMetrics filters out:
Thus, running your A/B tests with SplitMetrics, the platform won’t let you fill your experiments with absolutely irrelevant traffic thanks to built-in filters.
We already made it clear that the confidence level is one of the most important metrics which helps to determine when to finish your experiment. Yet, it’s not the only one. Here is the list of metrics to consider before finishing your A/B test:
SplitMetrics calculates this metrics automatically, so all you have to do is analyze.
I’d also recommend ensuring that your experiment lasted for 5-10 days before finishing it.
This way, you’ll capture users’ behavior during all weekdays making the results even more accurate.
Once you finish your experiment, the fun part begins. You find out whether your hypothesis was wrong or right and try to find reasons behind the obtained results.
Above all, it’s incredibly important to understand that there’s no such thing as negative results of A/B testing. Even if your new design suffers a crushing defeat from the old one, the experiment prevents mindless upload of these new creatives to the store. Thus, an A/B test with negative results averts mistakes that could cost your app thousands of lost installs.
There’s one more metric we should consider before deciding to use a winning variation in the App Store.This metric is called ‘Improvement’, it shows increase or decrease in the conversion rate of the variations in comparison to the control one.
If an A/B experiment shows that your new design wins by 1% or less, don’t rush to upload it to the store straightway. Remember that only the advantage of at least 3-4% can really impact the conversion of your app’s store product page.
There are cases when publishers draw quality traffic to their experiments but the confidence level refuses to grow. It normally happens because of a similar performance of the variations due to weak hypotheses. Keep in mind that even if your variations look totally different, it doesn’t prove the strength of your hypothesis. In such a situation, it’s highly recommended to finish the experiment and revise creatives.
Prepare yourself to the fact that your assumptions will be wrong as a rule. Yet, finding a game-changing product page layout definitely worth the effort.
Sustainable results that change your conversion rate for the better is the ultimate goal of experimenting with your app’s product page unless you are an A/B testing enthusiast and run such tests for sheer delight.
Many publishers wonder how to measure the impact of test results in the main stores. It is indeed an interesting yet tricky question. For sure, you should have a finger on the pulse of your conversion fluctuation after the upload of a new product page version.
Nevertheless, remember that any app store is a compound ever-changing environment teeming with factors influencing your app’s performance. To see a positive impact of your A/B testing results you should always be consistent.
For instance, let’s imagine you tested your new screenshots on users from Hong Kong. You got stunning results and uploaded them to the US store, as it’s your core market. You expect the same conversion boost you saw during the test but nothing really happens. It’s easy to claim A/B testing worthlessness in this situation, but targeting inaccuracy is to blame here.
The same is true when you apply the results of testing in the Google Play to the App Store failing to acknowledge the difference between Android and iOS users, their unlike perception of the store, varying behavioral patterns, etc.
The main recommendation here is to be diligent and consistent. Test only 1 element and only 1 change at a time as experimenting with different concepts at once, you will probably get ambiguous results requiring multiple follow-up tests. Then you’ll see app’s performance improvement in iTunes or Google Console without extra effort.
Providing you are ready to take time and use your wits, split-testing testing will become a safeguarded tool in your ASO arsenal. A/B experiments don’t only boost apps’ performance on both paid and organic traffic, they also provide essential metrics and analytics which can and should be applied in your audacious marketing activity.