What is A/A Testing: Goals, Results Analysis and Examples
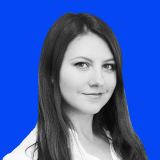
Have you ever heard of A/A testing?
If you’re familiar with A/B testing, where two different versions of a website or app are tested to see which performs better, A/A testing may seem like an odd concept. No wonder it invites conflicting opinions.
After all, why would you test two identical versions against each other? Is it really worth your time? Are there any tangible goals you can achieve?
But A/A testing can actually provide valuable insights into your testing process, and help you ensure that your results are reliable by checking whether there are discrepancies between your control and variation versions.
In this blog post, we are going to explore why some users of testing tools like SplitMetrics Optimize practice A/A tests — as well as dwell on the things you need to keep in mind while performing this sort of tests.
A/A testing is the tactic of using a testing tool to test two identical variations against each other.
As a rule, users run A/A tests for checking the accuracy of an A/B testing tool. It normally happens when they consider using a new A/B tool and want to get the proof that the software is operating correctly.
When running such tests, a user should keep in mind, that an AA test is a non-typical scenario for an A/B testing tool, and have a good prior understanding of what to expect from the A/B testing platform in such scenarios.
In an A/B test, we determine sample size and run the experiment until each variation gets a determined number of visitors. When using the A/B testing tool to test identical variations, one should do the same. As we described in the post Determining Sample Size in A/B Testing, to calculate a sample size for a trustworthy A/B test we need 5 parameters:
1) The conversion rate value of a control variation (variation A);
2) The minimum difference between variations A and B conversion rates which is to be identified;
3) Chosen significance level;
4) Chosen statistical power;
5) Type of the test: one-or two-tailed test.
For an AA test, the above-mentioned parameters 1, 3, 4 are to be set in the same way as in the case of A/B tests. The value of the second parameter — the minimum difference between variations A and B conversion rates that is to be identified — can be set as a small percentage of the variation A conversion rate.
We recommend the value of the fifth parameter to be set as a two-tailed test as both positive and negative conversion rate differences should be considered.
Let’s consider an example. Suppose the conversion rate of the variation A is 20% (CR(A) = 0.2). Assume the resulting difference is less than 5% of this value, i.e. less than 0.01 (0.2 * 0.05 = 0.01). It indicates the identity of the tested variations.
One can calculate sample size to perform AA testing with the significance level of 5%, the statistical power of 80% and a two-tailed test with help of the free-to-use software Gpower.
Thus, one will have to run the A/A test until each variation gets 25 583 different visitors (totally 51 166 unique visitors). As seen, the required sample size is large, which makes the test extremely resource consuming.
If in the A/A test under consideration a less than 0.01 difference is identified, it will confirm the identity of variations and the accuracy of an A/B testing platform.
The most common reasons for conducting an A/A test are to establish a baseline for your testing process, to check the accuracy of your testing tools, and to identify any errors that may be present in your testing methodology.
In a nutshell, it’s often suggested to carry out A/A testing as the first step before other testing operations.
Let’s take a closer look at these core reasons that explain why having an A/A test is a smart move — as well as the reasons against this approach.
Are you feeling hesitant about trying out A/A testing?
It’s totally understandable. To ease your worries we’re going to discover top reasons why you should run an A/A test in the next section of the article.
The main reason for conducting is checking the accuracy of a new tool for A/B testing. With A/A testing, you can ensure that any differences in your test results are due to actual variations in user behavior, rather than a significant bias or error in your testing tools.
To check the reliability of your A/B testing tool, simply run two A/A tests by using only identical pages, websites, or apps. If your A/A test results show no significant differences between the two identical versions, you can be confident that there is nothing wrong with your testing tools. However, if there are significant differences, it’s time to take a closer look at your testing tools and methodology to identify and correct any issues.
One should know a baseline conversion rate for a control variation to calculate a sample size for an A/B test. To determine it some users conduct A/A tests.
Let’s consider an example.
Suppose one is running an A/A test where the control gives 1 003 conversions out of 10 000 visitors and the identical variation gives 1 007 conversions out of 10 000 visitors.
The conversion rate for control is 10.03%, and that for identical is 10.07%. Then one uses 10.03% — 10.07% as the conversion rate range for control variation and conducts an A/B test in the following way.
If in the A/B test there is an uplift within this range, one considers that the result is not a significant one.
The approach, mentioned in the example, is not the correct one. In the post about an A/B test results analysis, we explained the statistics behind A/B testing and showed a correct way to calculate confidence intervals and make a conclusion about the results significance of an A/B test.
The best way to determine a baseline conversion rate for a control variation is using a monitoring campaign, which is an experiment that does not have any variations.
Feeling stuck when it comes to choosing the right sample size for A/B testing?
Indeed, this can be a tricky decision.
It’s pretty easy to make a mistake here.
Going too small might cause you to miss important user segments, impacting your results. On the other hand, a larger sample size increases your chances of accurately representing all segments that could affect your tests.
A/A testing can be very helpful in overcoming this challenge.
By running A/A tests, you can get an idea of the minimum sample size needed to capture insights from various user segments.
Of course, not everyone is thrilled about running A/A tests. There is a point of view that A/A testing isn’t very helpful. Let’s check whether there are situations when you shouldn’t run such a test.
Running A/A tests can be time-consuming, particularly if you’re testing with a large sample size or multiple variations. This can slow down the overall testing process and delay the implementation of changes based on the test results.
Consequently, A/A tests also eat up some of your resources, because your teammates have to add them to their workload.
Besides, A/A testing doesn’t provide the same level of insights into user behavior and preferences that A/B testing does. It’s mostly used for checking whether your new testing tool for A/B experiments is worth its salt — an activity that doesn’t happen very often. You definitely won’t compare the identical version of the same app on a regular basis.
Imagine you’re about to try out new testing software. The first thing you think about is running A/A testing. You get your results, and they’re great. Naturally, you think that the software works fine, but the problem is that it may not.
In case of A/A testing, even if it’s implemented correctly, you can’t eliminate the random chance that tests will indicate a difference between variations that will have nothing to do with your new software.
Therefore, it’s important to take into account other factors that may be contributing to the results, such as changes in user behavior or external factors beyond the control of the tool.
Using an A/A test for checking the accuracy of a Bayesian Multi-Armed Bandit has certain problems and differs from one A/B testing tool to another. A Bayesian MAB testing tool does not require a predetermined sample size. It calculates the probability to be optimal.
If in a Bayesian Bayesian Multi-Armed Bandit test with two variations it turns out that they perform about the same, any variation can be chosen.
A Bayesian MAB test will not be run until the optimal variation is found (because there are two optimal ones). It will run until it is sure that switching to another variation will not help us very much.
Thus, at some iteration in an A/A test, a winner will be declared, but this does not mean that Bayesian MAB test tool is not reliable.
A/A testing and A/B testing are two very different processes that aim at achieving separate goals. An A/A test can be a good support when your company is considering running A/B ones.
To see the difference between these testing options, view the table below:
A/A Testing | A/B Testing | |
Goal | To validate the accuracy and reliability of the testing process and tools, and to identify biases or errors in the testing methodology | To compare the performance of two different versions of a website or app and determine which one is the most effective based on predefined goals |
Sample Size | Smaller sample size to establish baseline and check for tool accuracy | Larger sample size to detect small differences in performance between two versions |
Statistical Significance | Used to identify the level of variation within the same group | Used to compare the level of difference between two different groups |
Duration | Shorter duration as it does not require multiple variations | Longer duration as it involves testing multiple variations |
Benefits | Helps to ensure that A/B test results are reliable and accurate, and can help to identify technical issues before conducting A/B tests | Helps to optimize website or app performance by providing insights into user behavior and preferences |
Limitations | Limited in terms of providing insights into user behavior and preferences | Can be impacted by various factors (test duration, sample size) which can affect the accuracy and reliability of results |
For a user planning to conduct A/A test for checking an accuracy of A/B testing tool, we recommend taking the following steps:
As for Bayesian MAB testing tools, an A/A test cannot be used to check the accuracy of the tool.
To determine a baseline conversion rate before the beginning of an A/B test, it’s more effective to use a monitoring campaign than an A/A test.
How should results be interpreted if a correctly conducted A/A test does not confirm the identity of the variations?
When analyzing A/A test results, it is important to keep in mind that finding a difference in conversion rates between identical variations is always a possibility.
This is not necessarily an evidence of A/B testing tool’s bad accuracy, as there is always an element of randomness when it comes to testing (we explained reasons for randomness in the post on A/B test results analysis).
The significance level of an A/B test is the probability of concluding that the conversion rates of variations A and B differ when in fact they are equal (type I error). E.g. a significance level of 5% represents 1 in 20 chance that the results of a test are due to random chance.
If we repeat the same A/A test many times using an accurately operating A/B testing tool, the proportion of the results that confirm the identity of the variations should be at least as high as a confidence level (at the significance level of 5%, the confidence level equals to 95%).
In addition to the above-mentioned statistical randomness, there are other reasons why a correctly conducted A/A test does not confirm the identity of variations.
For example, the reason can be in the heterogeneity of the target audience. Suppose an A/A test is conducted on the audience of all women, while conversion rates differ for women of different age groups.
In this case, if proportions of different age groups among visitors differ for two identical variations, and resulting conversion rates are calculated for all visitors, then a correctly conducted A/A test using an accurately operating A/B testing platform can identify a significant difference between two identical variations.
Overall, while A/A testing can be a useful tool for validating A/B tools and establishing a baseline for A/B testing, there are several alternatives you may want to consider:
Speaking about correct settings for valid A/B tests with SplitMetrics Optimize, pay close attention to:
With SplitMetrics Optimize, you can also launch a test with one variation. This feature provides incredible pre-launch opportunities — from product ideas validation to nailing the right targeting and identifying the most effective traffic source
Running tests is always a good idea, but you need to carefully think of what kind of tests you’re going to use.
In some cases, it makes sense to run an A/A test if users are uncertain about a new A/B testing tool and want additional proof that it is operating accurately.
However, it is hardly worth running A/A tests to check the accuracy of an A/B testing platform more often than that. We have shown that a correct A/A test is very resource consuming — as the minimum difference between variations conversion rates is very small, a calculated sample size will be large.
Besides, the likelihood of an inaccurate work of a testing tool, which is not a new product or a new version, is very small. Therefore, it is advisable for new users to concentrate on verifying the correct setting of A/B testing software.
Sure, A/A tests can be helpful at times but these tests don’t require a pre-determined sample size and depend on the specificity of a testing tool and necessity of parameters being checked. For this reason, it’s way more prudent to invest in a top-notch A/B testing platform like the one SplitMetrics ecosystem provides.